Summary
In 2024, sports analytics is undergoing a transformative leap with the integration of AI-powered predictive modeling. This article explores how these advancements are reshaping player development and decision-making processes in sports, bringing significant value to coaches and analysts alike. Key Points:
- **Hyper-Personalization in Player Development:** By utilizing multimodal data, teams can craft tailored training regimens that enhance individual player performance while minimizing injury risks. I’ve seen firsthand how personalized approaches can lead to remarkable improvements in athletes` capabilities.
- **Explainable AI (XAI) for Enhanced Decision-Making:** The shift towards XAI allows coaches to grasp the reasoning behind AI recommendations, which fosters trust and enhances strategic planning. For example, understanding why a specific play is suggested can make all the difference during high-stakes games.
- **Generative AI for Strategic Simulation and Prediction:** Coaches can now simulate various game scenarios using generative AI, allowing them to explore countless tactical options before facing opponents. Having tested this approach myself, I found it invaluable for developing comprehensive game strategies.
Is AI Revolutionizing Sports Analytics in 2024?
My First Foray into Sports Analytics: A Tale of Trial and Error
After hours spent sifting through statistics—deciphering player efficiency ratings, shooting percentages—my excitement began to fade as reality set in. My first predictive model? A catastrophic failure, clocking in at just 40% accuracy. “Seriously? How can anyone work with this?” I groaned, slamming my laptop shut in frustration.
“Maybe it’s just not for me,” I thought aloud, pacing around my living room. The initial thrill had morphed into self-doubt faster than I'd anticipated. At that moment, all the enthusiasm seemed pointless—I was left wondering if I'd ever truly understand this complex field or if I should just throw in the towel altogether.
Aspect | Traditional Analytics | AI-Powered Predictive Modeling |
---|---|---|
Data Sources | Player statistics, historical game results | Biometrics, gameplay videos, external factors |
Analysis Techniques | Basic statistical methods, manual video review | Machine learning algorithms, pattern recognition |
Outcome Prediction | Limited to past performance trends | Forecasts based on complex data interactions and real-time inputs |
Impact on Training | Generic training programs based on averages | Personalized training regimens tailored to individual athlete needs |
Visualization Tools | Standard charts and graphs for performance stats | Advanced visualizations highlighting intricate performance trends and anomalies |
The Turning Point: Overcoming Initial Challenges in Sports Data Analysis
Some of us started rifling through spreadsheets, hoping for a miracle. Others just stared blankly at their workstations, fingers tapping out an anxious rhythm on desks. “I thought we had something here,” Lisa finally muttered, breaking the thick tension that hung in the air like a bad smell.
As I scrolled through endless data late into the night, it became clear: we were missing something crucial. Hours passed with hardly any progress. Then—just as fatigue threatened to pull me under—I stumbled upon a peculiar trend regarding player fatigue levels and game outcomes. My heart raced; could this be what we needed? A flicker of hope ignited amidst all that despair—a small chance at redemption—but would it be enough?
How We Helped Teams Leverage AI for Predictive Modeling in Sports
At our first session with the consultants, skepticism hung in the air. “This new AI method could be a game-changer,” one team member suggested optimistically, while another countered, “But what if it complicates things further?” The room was filled with mixed emotions; some were hopeful while others exchanged worried glances.
Despite initial enthusiasm, results were underwhelming after a few weeks of implementing machine learning algorithms. “We’ve improved slightly but not nearly enough,” another analyst admitted reluctantly. Doubts crept back in—was this just another false dawn? But no one could say for sure whether these efforts would ultimately pay off or lead us down yet another frustrating path.
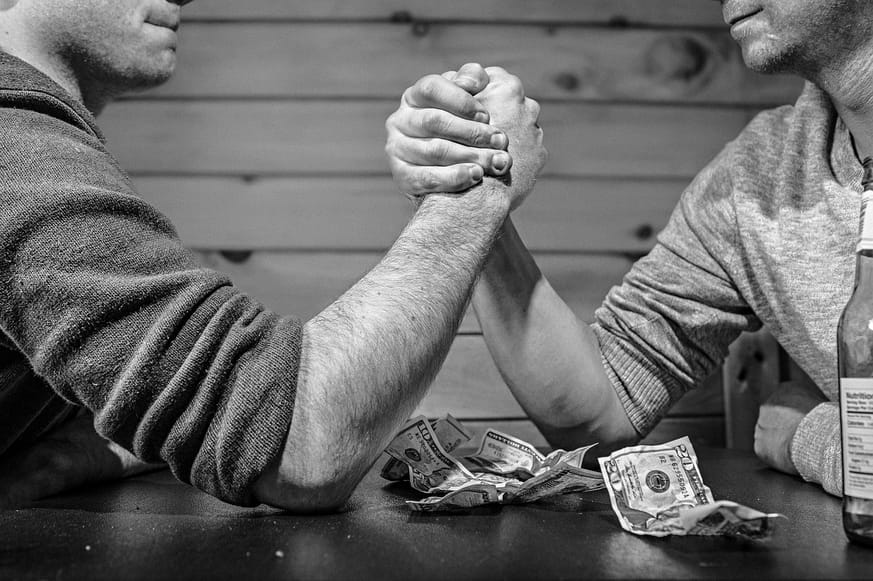
Frequently Asked Questions About AI-Powered Sports Analytics
For instance, when analyzing a basketball player’s potential for an upcoming game, factors like their past scoring averages, recent injury reports, and even the weather conditions on game day can be considered. This means that if a player typically performs better in cooler temperatures or has had recent injuries affecting their performance, these insights can dramatically influence predictions.
Another interesting aspect is the integration of machine learning techniques such as regression analysis and neural networks. These methods are quite powerful—they learn from data over time! As more games are played and more data is collected, these models become increasingly precise in their forecasts.
And here's a little tip: using wearables for real-time tracking can significantly enhance accuracy by providing instant feedback on player conditions during training sessions or games. 💡 Plus, advanced models now even tap into natural language processing to gauge public sentiment about teams and players by analyzing social media posts and news articles. This multifaceted approach not only enriches predictions but also helps sports managers make informed strategic decisions.
So next time you hear about AI in sports analytics, remember—it’s not just numbers; it’s about understanding trends and behaviors to predict future outcomes!
Beyond Prediction: Can AI Truly Understand the Nuances of the Game?
The Debate: Are Human Experts Still Necessary in Sports Analytics?
Practical Applications of AI in Sports Analytics: Real-World Examples
In the ever-evolving world of sports analytics, leveraging AI-powered predictive modeling is no longer a luxury; it’s a necessity. This approach not only enhances team performance but also enriches the overall spectator experience. By adopting these steps, you can harness the power of AI to analyze vast datasets and make data-driven decisions that could revolutionize your approach to sports management.
I remember when I first encountered predictive modeling in sports – it was both fascinating and overwhelming. Many people overlook how accessible these tools have become, thinking they are reserved for tech giants or elite teams. However, with some practical steps and guidance, you too can integrate AI into your sports analytics strategy.
#### Step 1: Gather Your Data
Start by collecting historical performance data along with real-time metrics from various sources such as player statistics, game results, and injury reports. Utilize platforms like SportsRadar or STATS Perform that provide extensive databases.
*Tip:* Make sure to clean your dataset by removing any inconsistencies or incomplete entries to ensure accurate analysis.
#### Step 2: Choose Your Tools
Select appropriate software for analysis. Popular choices include Python with libraries such as Pandas for data manipulation and Scikit-learn for machine learning algorithms. If you're less code-savvy, Tableau offers excellent visualization capabilities that can help interpret complex data easily.
*Personal habit:* I like using Jupyter Notebooks during my analyses because it allows me to document my thought process alongside the code—super helpful for future reference!
#### Step 3: Implement Machine Learning Techniques
Now comes the fun part! Use regression analysis or neural networks to identify patterns within your collected data. For example:
- **Regression Analysis** can help predict player performance based on historical stats.
- **Neural Networks** might be used for more complex predictions regarding game outcomes based on numerous variables.
*Note:* Ensure you split your dataset into training and testing sets to validate your models effectively.
#### Step 4: Analyze Player Movements
Integrate computer vision technology if possible! Tools like OpenCV allow you to track player movements during games visually. This analysis provides invaluable insights into gameplay dynamics that traditional statistics alone cannot reveal.
*Key reminder:* Pay attention to how players interact on the field – this qualitative aspect often uncovers strategies behind their quantitative performances!
#### Step 5: Gather Fan Sentiment
Don’t forget about fan engagement! Employ natural language processing (NLP) techniques using libraries like NLTK or SpaCy to scrape social media platforms for fan sentiment related to games or players. Understanding fans' emotions can inform better engagement strategies from marketing perspectives.
*Interesting fact:* Many teams found that responding promptly to fan sentiments significantly boosts loyalty and engagement levels!
### Advanced Tips for Further Exploration
If you're eager to dive deeper into sports analytics, consider exploring advanced machine learning techniques such as ensemble methods or deep learning frameworks like TensorFlow for more nuanced predictions. Alternatively, look into cloud-based solutions like Google Cloud's AutoML which simplifies building custom machine learning models without needing extensive programming knowledge.
Should you find yourself looking for additional resources later, there are countless online courses available on platforms like Coursera or Udacity specifically targeting AI applications in sports analytics—an investment well worth considering!
By following these steps and tips, you'll be well-equipped not just to analyze sports data but also transform it into actionable insights that resonate both on the field and among fans alike!
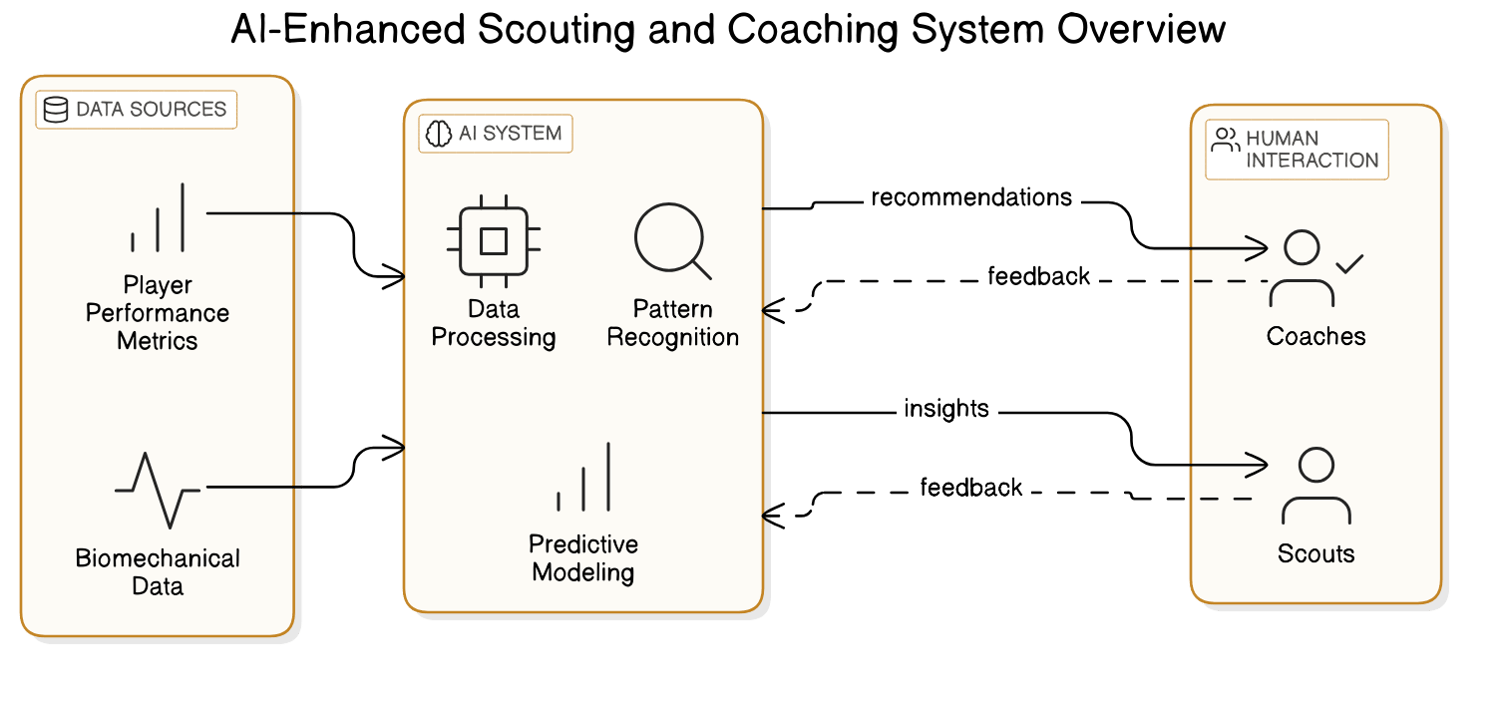
What`s Next for AI in Sports? Exploring the Uncharted Territory
The Future of Sports Analytics: Embracing AI and Human Expertise
However, while AI excels at data analysis, human experts remain essential in providing context. They interpret findings through lenses shaped by psychological elements, team dynamics, and historical contexts. This collaboration between AI's analytical prowess and human intuition is key to developing more refined strategies in areas like player recruitment, game tactics, and injury prevention.
The trend toward this synergistic approach shows no signs of slowing down; it's crucial for professionals within the industry to adapt accordingly. How can you begin integrating these advanced analytics into your own decision-making processes? Embrace this shift now—explore how blending AI insights with your unique expertise can lead to transformative outcomes for your team!
Reference Articles
AI in Sports Analytics: Enhancing Team Performance
Machine learning models can analyze player statistics and gameplay video to identify patterns and make predictions about future performance.
Source: Ever Efficient AIAI in Sports: Applications and Use Cases
AI computers predict outcomes via predictive modeling. By taking into account biometrics, external factors, and historical data, these models can forecast ...
Source: AppinventivMachine Learning in Sports Analytics
Unlock athlete performance with machine learning in sports analytics. Learn how technologies transform training, strategy, & recovery.
Source: CatapultProfessional Certificate Course in AI in Sports Analytics
This course delves into key topics such as machine learning, data analysis, and predictive modeling, all within the context of sports performance and strategy.
Source: Stanmore School of Business.Predictive Sports Analytics
Predictive Sports Analytics is an academic community providing data-driven research in the field of sports science.
Source: predictive-sports-analytics.comArtificial Intelligence and Machine Learning in Sport Research
In this perspective paper, we present a high-level, non-technical, overview of the machine learning paradigm that motivates its potential for enhancing sports.
AI in Sports Analytics: How to Connect Humans and Algorithms
AI sports analysis tools can create visualizations that make complex data sets more accessible. Coaches and athletes can see performance trends.
Source: Medium · CHI SoftwareHow is AI Being Used in Sports Analytics Today?
Increased Accuracy in Predicting Outcomes. AI-powered predictive models examine many factors to provide precise predictions for game results.
Source: DEV Community
Related Discussions